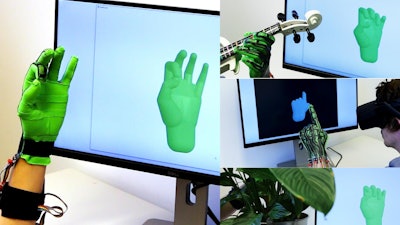
Capturing interactive hand poses in real time and with realistic results is a well-examined problem in computing, particularly human-centered computing and motion capture technology.
Human hands are complex--an intricate system of flexors, extensors, and sensory capabilities serving as our primary means to manipulate physical objects and communicate with one another.
The accurate motion capture of hands is relevant and important for many applications, such as gaming, augmented and virtual reality domains, robotics, and biomedical industries.
A global team of computer scientists from ETH Zurich and New York University have further advanced this area of research by developing a user-friendly, stretch-sensing data glove to capture real-time, interactive hand poses with much more precision.
The research team, including Oliver Glauser, Shihao Wu, Otmar Hilliges, and Olga Sorkine-Hornung of ETH Zurich and Daniele Panozzo of NYU, will demonstrate their innovative glove at SIGGRAPH 2019, held 28 July-1 August in Los Angeles. This annual gathering showcases the world's leading professionals, academics, and creative minds at the forefront of computer graphics and interactive techniques.
The main advantage of their stretch-sensing gloves, say the researchers, is that they do not require a camera-based set-up--or any additional external equipment--and could begin tracking hand poses in real time with only minimal calibration.
"To our best knowledge, our gloves are the first accurate hand-capturing data gloves based solely on stretch sensors," says Glauser, a lead author of the work and a PhD student at ETH Zurich. "The gloves are soft and thin, making them very comfortable and unobtrusive to wear, even while having 44 embedded sensors. They can be manufactured at a low cost with tools commonly available in fabrication labs."
Glauser and collaborators set out to overcome some persisting challenges in the replication of accurate hand poses. In this work, they addressed hurdles such as capturing the hand motions in real time in a variety of environments and settings, as well as using only user-friendly equipment and an easy-to-learn approach for set-up. They demonstrate that their stretch-sensing soft gloves are successful in accurately computing hand poses in real-time, even while the user is holding a physical object, and in conditions such as low lighting.
The researchers utilized a silicone compound in the shape of a hand equipped with 44 stretch sensors and attached this to a glove made of soft, thin fabric. To reconstruct the hand pose from the sensor readings, the researchers use a data-driven model that exploits the layout of the sensor itself. The model is trained only once; and to gather training data, the researchers use an inexpensive, off-the-shelf hand pose reconstruction system.
For the study, they compare the accuracy of their sensor gloves to two state-of-the-art commercial glove products. In all but one hand pose, the researchers' novel, stretch-sensing gloves received the lowest error return for each interactive pose.
In future work, the team intends to explore how a similar sensor approach could be used to track a whole arm to get the global position and orientation of the glove, or perhaps even a full body suit. Currently the researchers have fabricated medium-sized gloves, and they would like to expand to other sizes and shapes.
"This is an already well-studied problem but we found new ways to address it in terms of the sensors employed in our design and our data-driven model," notes Glauser. "What is also exciting about this work is the multidisciplinary nature of working on this problem. It required expertise from various fields, including material science, fabrication, electrical engineering, computer graphics, and machine learning."