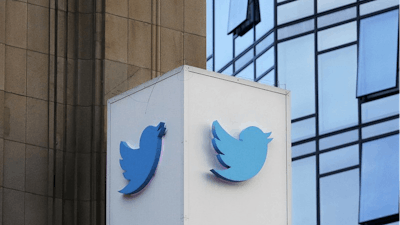
Researchers at Caltech have demonstrated that machine-learning algorithms can monitor online social media conversations as they evolve, which could one day lead to an effective and automated way to spot online trolling.
The project unites the labs of artificial intelligence (AI) researcher Anima Anandkumar, Bren Professor of Computing and Mathematical Sciences, and Michael Alvarez, professor of political science. Their work was presented on December 14 at the AI for Social Good workshop at the 2019 Conference on Neural Information Processing Systems in Vancouver, Canada. Their research team includes Anqi Liu, postdoctoral scholar; Maya Srikanth, a junior at Caltech; and Nicholas Adams-Cohen (MS '16, PhD '19) of Stanford University.
"This is one of the things I love about Caltech: the ability to bridge boundaries, developing synergies between social science and, in this case, computer science," Alvarez says.
Prevention of online harassment requires rapid detection of offensive, harassing, and negative social media posts, which in turn requires monitoring online interactions. Current methods to obtain such social media data are either fully automated and not interpretable or rely on a static set of keywords, which can quickly become outdated. Neither method is very effective, according to Srikanth.
"It isn't scalable to have humans try to do this work by hand, and those humans are potentially biased," she says. "On the other hand, keyword searching suffers from the speed at which online conversations evolve. New terms crop up and old terms change meaning, so a keyword that was used sincerely one day might be meant sarcastically the next."
Instead, the team used a GloVe (Global Vectors for Word Representation) model to discover new and relevant keywords. GloVe is a word-embedding model, meaning that it represents words in a vector space, where the "distance" between two words is a measure of their linguistic or semantic similarity. Starting with one keyword, this model can be used to find others that are closely related to that word to reveal clusters of relevant terms that are actually in use. For example, searching Twitter for uses of "MeToo" in conversations yielded clusters of related hashtags like "SupportSurvivors," "ImWithHer," and "NotSilent." This approach gives researchers a dynamic and ever-evolving keyword set to search.
But it is not enough just to know whether a certain conversation is related to the topic of interest; context matters. For that, GloVe shows the extent to which certain keywords are related, providing input on how they are being used. For example, in an online Reddit forum dedicated to misogyny, the word "female" was used in close association with the words "sexual," "negative," and "intercourse." In Twitter posts about the #MeToo movement, the word "female" was more likely to be associated with the terms "companies," "desire," and "victims."
The project was a proof-of-concept aimed at one day giving social media platforms a more powerful tool to spot online harassment. Anandkumar's interest in the topic was intensified by her involvement in the campaign to change the shorthand name of the Neural Information Processing Systems conference from its original acronym, "NIPS," to "NeurIPS."
"The field of AI research is becoming more inclusive, but there are always people who resist change," says Anandkumar, who in 2018 found herself the target of harassment and threats online because of her successful effort to switch to an acronym without potentially offensive connotations. "It was an eye-opening experience about just how ugly trolling can get. Hopefully, the tools we're developing now will help fight all kinds of harassment in the future."