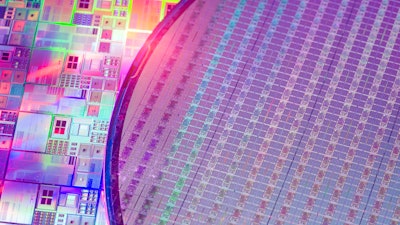
People applying the principles of neuromorphic engineering in their work develop devices or components that mimic the human brain’s functionality and structure. Ongoing research in this area — often referred to under the broader umbrella of brain-inspired computing — suggests this focus could lead to critical advancements in microprocessors, artificial intelligence and more. What should you expect regarding the impact of neuromorphic engineering on your projects?
Increased Direction for Design Decisions
Using neuromorphic engineering to design a new microprocessor or other component is still an emerging option. Many people must try never-before-attempted options, embracing an innovative spirit while hoping for the best. However, that could change before long.
A joint effort between researchers at Tohoku University and the University of Cambridge resulted in a reference map that could give valuable guidance to designers and other professionals. The teams focused on organic electrochemical transistors, which people often use to control ionic movement in neuromorphic engineering. One of the critical findings was that an ion’s response time depends on its size.
The researchers combined the findings of numerous experiments to create a map of rational design guidelines relating to ion size and the composition used for the active layer of a neuromorphic device. They believe further research will increase the possible applications for artificial neural networks and aid designers in finding better-conducting polymers as they make material-related choices.
All neural networks have at least three layers, with one or more used for information processing. Some designers have gotten increasingly creative with the materials they use for neuromorphic projects. Efforts have involved fungi, honey and collagen, to name a few. Design professionals with more guidance regarding the likely outcomes of their decisions feel increasingly confident and eager to explore.
Shortened Training Time Frames
Another impact of neuromorphic engineering that could benefit designers is the ability to train advanced algorithms in less time. Many tech experts have explored options for on-device training to save energy and improve data security.
These alternatives allow embedded components to become more effective as people use them. In one case, researchers developed a process requiring only a quarter-megabyte of memory. Another advantage is that the data stays on the device, resulting in tighter security since there’s no need to send the information for external processing.
A recent case that could change neuromorphic engineering involved creating a smart biosensor that works without external training platforms or techniques. The proof of concept could diagnose cystic fibrosis through sweat samples.
This work solves multiple problems because conventional ways of training neuromorphic chips are energy-intensive and time-consuming. Experiments also indicated the researchers could apply their neuromorphic chips to new use cases, even after training them.
The reduced training time also gives more time for other design phases, such as testing. Many designers in numerous fields subject their creations to vibration testing. This approach can identify defects or verify intended functionality.
Microprocessors typically undergo structural testing that shows whether they’ll perform as expected during real-life usage. Designers must identify the reasoning behind shortcomings and make strategic decisions for targeted improvements. An accelerated training period allows more time for testing and changes when necessary.
Reduced Hardware Costs and Better Scalability
Many people working to design microprocessors agree the impact of neuromorphic engineering on their projects could be substantial. However, they recognize there are still many challenges to overcome. One relates to how developing new, advanced hardware remains a costly endeavor. Relatedly, even if a design decision works well in the lab, uncertainties often remain regarding how scalable it would be in the real world.
However, researchers recently made remarkable progress in this area. They made neuromorphic hardware more cost-effective and scalable by co-integrating the synapses and single transistor neurons. The team did this on one wafer using standard silicon complementary metal-oxide-semiconductor (CMOS) technology.
The approach resulted in simplified, less expensive hardware that uses the same memory and logic transistors as mass-produced options. Microprocessors contain billions of transistors, so any improvement in that component could have long and far-reaching effects.
In another example that could make neuromorphic hardware more scalable, researchers built a circuit that mimics the human brain’s synaptic activity. It can transmit and receive information using only single photons. The researchers clarified they must do further work to test their innovation. However, they believe it could result in artificial neural networks that work 100,000 times faster than human brains while overcoming known challenges of neuromorphic engineering.
Broadened Applications for Microprocessor Components
The increasing impact of neuromorphic engineering on modern designs has also encouraged people to explore new ways to utilize the components used when making microprocessors. One instance involved a team creating a new material for memristors, which handle logic-based tasks and storage needs.
The researchers made the memristors out of halide perovskite nanocrystals, which people have previously used in solar cells. Their inventions include a dual-operating mode affecting how the signal works. In one possible state, it gets progressively weaker and eventually nonexistent. Alternatively, the researchers could use the other mode for applications requiring a constant signal.
The researchers confirmed it was previously necessary to program memristors to operate in one of those modes. However, using them in more than one increases adaptability for different artificial neural network architectures.
Lab tests on 25 memristors involved using them to take 20,000 measurements, thereby simulating a computational problem on a complex network. Despite this progress, the researchers must optimize these memristors before using them in real-world designs. Even so, because they mimic the brain’s activity more accurately than previous attempts, they’ll be instrumental in showing designers new possibilities and increased opportunities.
The Impact of Neuromorphic Engineering Is Worth Watching
These are some of the many ways neuromorphic engineering will reshape designers’ work and expand what they can do. Many achievements will result in high-tech hardware beyond what people have previously imagined.
Ongoing interest and investment in this area means progress happens regularly. Design professionals should keep up with the latest developments, studying their effects and examining how the research could change their current or future workflows. Additionally, since neuromorphic engineering is an emerging field, people must keep open minds and be willing to do things differently if technological achievements indicate they can.
Emily Newton is the Editor-in-Chief of Revolutionized and an industrial writer who enjoys researching and writing about how technology impacts different industries.